Unlocking the Power of Data Labeling Tools
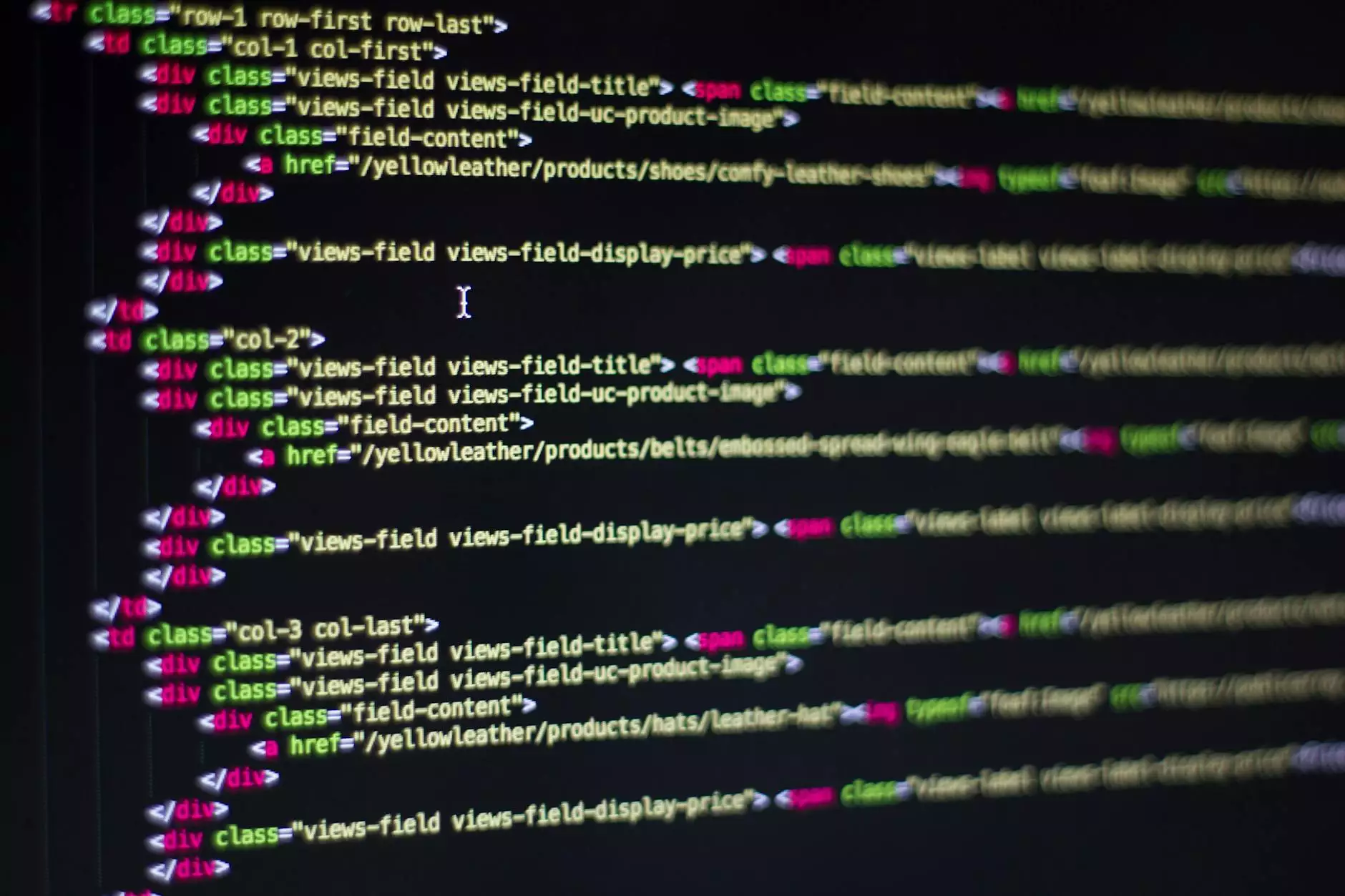
In the fast-evolving world of artificial intelligence (AI) and machine learning (ML), the process of data labeling has become a fundamental component of developing intelligent systems. The role of a data labeling tool cannot be overstated as it directly impacts the accuracy, efficiency, and overall effectiveness of ML models.
What is a Data Labeling Tool?
A data labeling tool is software designed to assist in the process of annotating and organizing data, primarily to train machine learning algorithms. Data labeling involves the identification and tagging of data (such as images, text, or audio) with relevant information that denotes its attributes or features. This allows ML models to learn from the data and make predictions or classifications based on new, unseen data.
The Importance of Data Annotation
Data annotation plays a crucial role in various AI applications, from image recognition to natural language processing. With the explosive growth of data generated every day, the demand for precise and efficient data annotation methods has surged. Here are some reasons why data annotation is essential:
- Quality Data: High-quality labeled data is critical for the success of any AI project. It ensures that the models trained on this data are reliable and accurate.
- Improved Performance: Well-annotated datasets allow for better training, leading to improved performance in real-world applications.
- Scalability: As projects grow in scope, scalable data labeling tools enable teams to keep up with increasing data volumes without sacrificing quality.
- Customization: Businesses can tailor labeling processes to fit their specific needs, ensuring that their unique data characteristics are recognized.
Types of Data Labeling Tools
There are various data labeling tools available, each designed to cater to different types of data and labeling requirements. Here's an overview of some popular types:
1. Image Annotation Tools
Image annotation tools allow users to label visual data. This is crucial for applications like image classification, object detection, and facial recognition. Features often include bounding boxes, polygons, and segmentation options.
2. Text Annotation Tools
Text annotation tools help annotate text data for applications such as sentiment analysis, spam detection, and named entity recognition. They typically focus on tagging parts of speech, sentiment labels, or entities within the text.
3. Audio Annotation Tools
With the rise of voice recognition technologies, audio annotation tools are becoming increasingly important. These tools assist in labeling audio segments, transcribing speech, and identifying sounds for machine learning applications.
Choosing the Right Data Labeling Platform
Selecting the proper data labeling platform can significantly affect the quality of your machine learning project. Here are several factors to consider when making your choice:
- Usability: A user-friendly interface can simplify the labeling process, especially in collaborative environments.
- Collaboration Features: Look for tools that support multiple users, facilitating teamwork and efficiency.
- Integration: The ability to integrate with existing workflows and tools (like data storage and processing systems) is essential for a smooth operation.
- Cost Effectiveness: Evaluate the pricing model offered by various platforms to ensure that it fits within your budget while providing necessary features.
- Quality Assurance: Choose tools that offer quality control mechanisms to maintain high standards in the data labeling process.
Benefits of Using Data Labeling Tools
Adopting a robust data labeling tool can provide numerous advantages for businesses involved in AI and ML:
1. Enhanced Efficiency
Automating the labeling process can save significant time and manpower, allowing teams to focus on more critical tasks, such as model training and evaluation.
2. Consistency and Accuracy
Automation and standardized processes reduce human error, ensuring that the labeling is consistent across the dataset, which is vital for building dependable models.
3. Speedy Turnaround
The right labeling tools can drastically reduce the turnaround time for data preparation, accelerating the time-to-market for your AI solutions.
Key Features to Look For in a Data Labeling Tool
When scouting for a data labeling tool, you'll want to identify features that align with your project requirements:
- Support for Multiple Data Types: Ensure that the tool can handle the type of data you are working with.
- Custom Labeling Schemes: The ability to define your labeling criteria can enhance the relevance of the data prepared for your models.
- Advanced Annotation Capabilities: Look for features like real-time collaboration, keyboard shortcuts for faster annotation, and customizable templates.
- Analytics and Reporting: Understanding labeling efficiency and quality metrics can provide insights for continuous improvement.
Real-World Applications of Data Labeling Tools
Data labeling tools are used across various industries, showcasing their versatility and necessity:
1. Healthcare
In healthcare, labeled datasets are crucial for training algorithms that assist in diagnosis, medical imaging analysis, and patient data management.
2. Automotive
The automotive sector uses data labeling tools for training self-driving cars, which rely on vast amounts of accurately labeled visual data for safe navigation and understanding of surroundings.
3. Retail
In the retail space, data labeling enhances customer experience through personalized recommendations and sentiment analysis from customer reviews.
Conclusion
In today's data-driven world, the importance of effective data labeling cannot be overstated. A robust data labeling tool is not only a facilitator of quality data but also a catalyst for innovation in AI and machine learning applications. As businesses increasingly rely on data to drive decisions and strategies, adopting the right tools will foster success. Explore your options today at Keylabs.ai, where cutting-edge data annotation solutions await you!
Frequently Asked Questions About Data Labeling Tools
What is the cost of a data labeling tool?
The cost can vary widely based on features, usage volume, and the specific data types handled. Many platforms offer subscription models based on usage or one-time fees for perpetual licenses.
Can data labeling tools be used for multiple data types?
Yes, many modern labeling tools support various data types, including images, text, and audio, making them versatile for different projects.
Is machine learning knowledge necessary to use data labeling tools?
While having an understanding of machine learning can be beneficial, most data labeling tools are designed to be user-friendly and accessible to users without a technical background.
How does automation improve the data labeling process?
Automation minimizes manual effort, increases labeling speed, and helps maintain high consistency and accuracy across datasets, thus enhancing overall efficiency.
Where can I learn more about data labeling and its tools?
Resources such as blogs, online courses, and documentation from data labeling service providers, like Keylabs.ai, can be excellent starting points for learning more.