Understanding Annotation in Machine Learning: A Key to Business Success
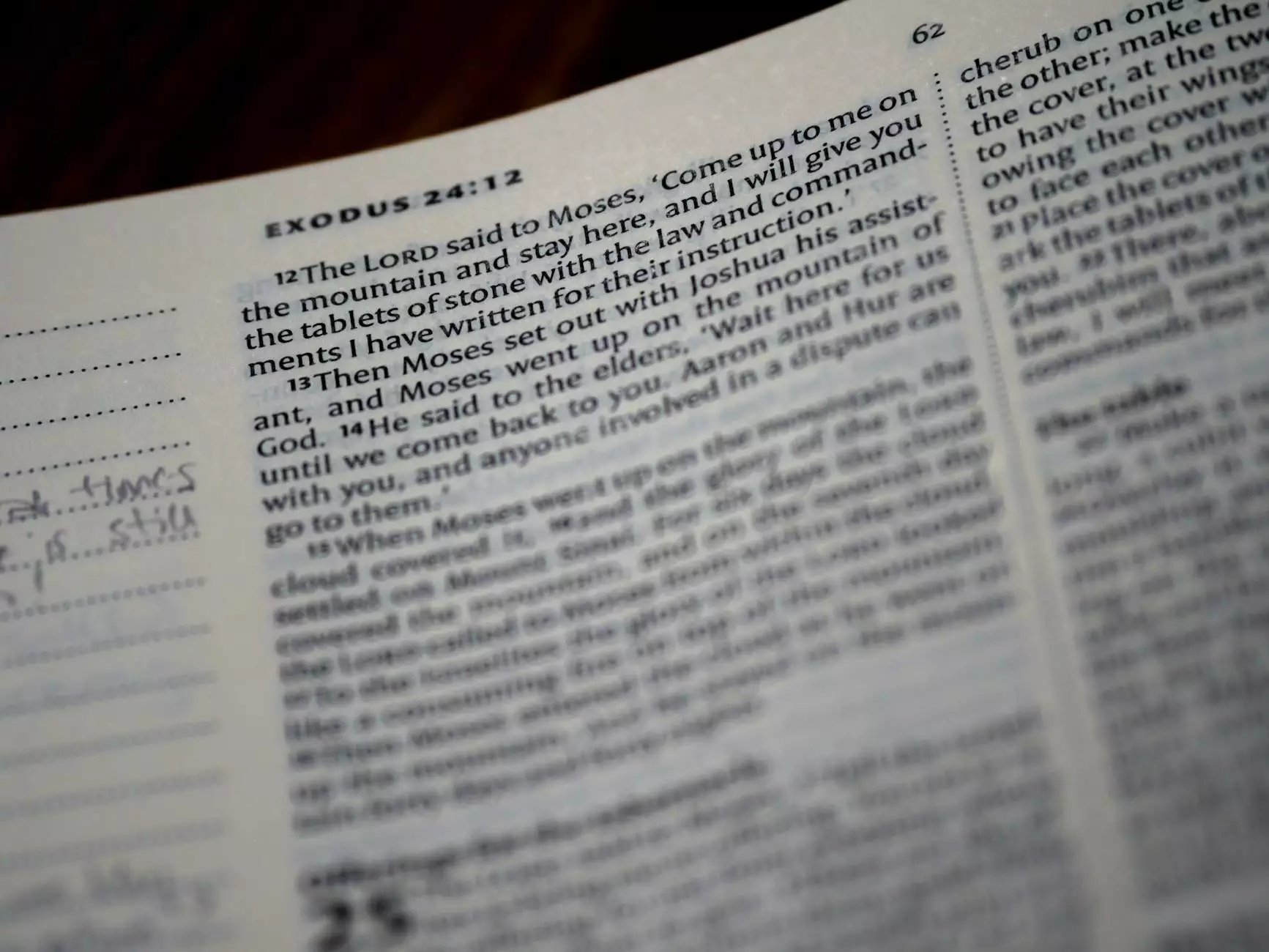
Machine Learning has become a pivotal technology for businesses today, and at its core, machine learning relies on data. However, the effectiveness of machine learning models significantly depends on the quality of the data fed into them. This is where annotation in machine learning comes into play. As organizations aim to improve their services—whether in home services, keys, or locksmiths—understanding the role of data annotation can provide a substantial competitive advantage.
The Role of Annotation in Machine Learning
Annotation in machine learning refers to the process of labeling data to make it understandable for machine learning algorithms. This data can range from images and videos to text and sounds. For instance, if a machine learning model is developed to identify different types of locks or key management systems, it must be trained using accurately annotated datasets.
Types of Data Annotation
There are several types of data annotation techniques that can be categorized based on the nature of the data. Here are some of the most common methods:
- Image Annotation: This involves labeling images with specific tags that describe the content within the image. For locksmith services, images of different types of locks can be annotated to help train models for recognition.
- Text Annotation: This approach is useful for extracting information from documents, user reviews, or emails, thereby improving customer relations and service delivery in home services.
- Video Annotation: This method allows for the tagging of moving images. In the locksmith industry, video data from surveillance footage can be used to train models to identify suspicious activities.
- Audio Annotation: Labeling audio files can help in understanding customer calls to improve service response times.
The Importance of Quality Annotation in Machine Learning
Quality in data annotation directly affects the performance of machine learning models. Poorly annotated data can lead to inaccurate model predictions, ultimately affecting business efficiency and client trust. Here are a few reasons why high-quality annotation is essential:
- Improves Accuracy: Well-annotated data ensures that the learning models understand the data correctly, leading to higher accuracy in predictions.
- Enhances Model Training: A model trained on correctly annotated data is more likely to generalize well to unseen data, which is crucial for businesses operating in dynamic environments like home services.
- Customer Satisfaction: For service-based businesses, understanding customer needs through accurate data helps in tailoring offerings, enhancing overall satisfaction.
How Annotation Supports Home Services and Locksmith Businesses
In the realm of home services and locksmiths, the integration of machine learning through effective data annotation can transform traditional operations. Here’s how:
1. Efficient Service Provision
Utilizing machine learning powered by annotated data can help locksmith companies streamline their operations. For example, machine learning algorithms can predict peak service hours based on historical data, leading to better staffing and inventory management.
2. Enhancing Customer Interaction
Text annotation of customer interactions can reveal patterns in customer queries and complaints. By analyzing this data, businesses can improve FAQ systems or chatbot responses, providing instant and accurate answers to potential customers.
3. Fraud Detection
For security-conscious customers, identifying fraudulent activities is paramount. By annotating video surveillance data, locksmith businesses can train AI systems to alert them of potential threats, enhancing customer trust and safety.
The Process of Data Annotation
Implementing an effective annotation strategy involves several steps:
Step 1: Define Objectives
Clearly outline what you want to achieve through machine learning. For locksmith services, this could be identifying different lock types or improving customer interaction through chatbots.
Step 2: Choose the Right Tools
Selecting the appropriate tools for data annotation is critical. Options include software for manual annotation, as well as automated tools that can accelerate the process.
Step 3: Annotate the Data
Once the tools are set, begin the annotation process. Depending on the data type, ensure that the labels are accurate and comprehensive.
Step 4: Quality Control
After annotation, it's essential to conduct quality checks. A team should review annotated data to verify accuracy, ensuring the highest standards are maintained.
Step 5: Model Training and Testing
Feed the annotated data into the machine learning model. Continuous testing and iteration based on model performance will refine the effectiveness of the solution.
Challenges in Data Annotation
Despite its importance, data annotation comes with challenges:
- Time Consuming: Annotating large datasets can be a labor-intensive process, requiring considerable time and resources.
- Subjectivity: Different annotators might have varying interpretations of what certain labels mean, leading to inconsistencies.
- Scale Issues: As businesses grow, the amount of data needing annotation can overwhelm existing systems.
Leveraging Outsourcing for Data Annotation
To overcome these challenges, many businesses opt to outsource data annotation to specialized service providers. Outsourcing can offer several advantages:
- Expertise: Professional annotators have the necessary skills and experience to ensure high-quality labels.
- Scalability: Outsourcing allows businesses to scale their data processing needs according to demand without overburdening existing staff.
- Cost-Effective: Utilizing external resources can often be more cost-effective than hiring in-house teams.
Future Trends in Machine Learning Annotation
The landscape of machine learning annotation is evolving, and several trends are emerging that will likely shape its future:
1. AI-Assisted Annotation
Increasingly, businesses are turning to AI tools to assist in the annotation process. These tools use pre-trained models to automate parts of the labeling process, improving efficiency dramatically.
2. Active Learning
This approach involves training a model with a small annotated dataset initially and then allowing it to identify labels for the next set of unlabeled data. This method maximally utilizes human resources while minimizing annotation needs.
3. Real-time Annotation
Real-time data annotation is essential for applications like autonomous driving or security systems. By implementing real-time annotation methods, businesses can improve responsiveness and accuracy instantly.
Conclusion
In summary, understanding annotation in machine learning is crucial for any business looking to leverage technology for competitive advantage. Particularly in the home services and keys & locksmiths industries, the integration of high-quality annotated data can lead to improved service provision, enhanced customer interaction, and robust operational efficiencies. As machine learning technologies continue to evolve, staying ahead of trends in data annotation will prepare businesses for future challenges and opportunities.
For businesses such as KeyMakr, adopting these strategies will not only optimize workflows but also enhance customer satisfaction and trust, ultimately driving growth in a rapidly changing environment.